Becoming Human: AI Progress
Jul 27, 2021 8406 seen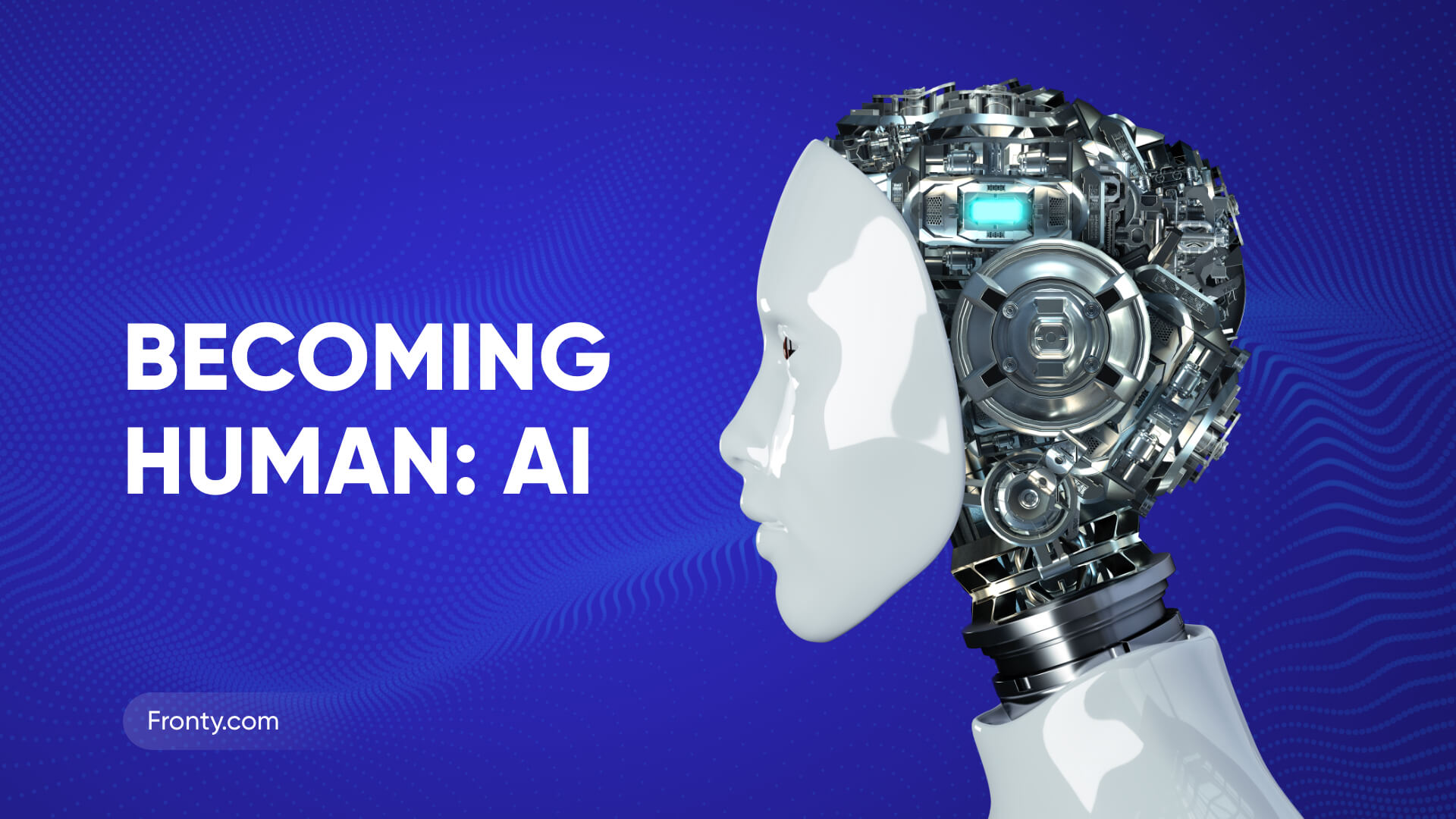
Artificial Intelligence Progress
People can acquire and apply general knowledge to solve problems in a wide range of subject areas. Some of them are absorbed by all people, such as walking and talking. Some people acquire specialized knowledge, usually as part of their profession, for example, surgeons, civil engineers, or truck drivers.
Deep Learning AI
Over the past five years, AI has made a huge impact. Not a day goes by without media coverage of AI applications, and start-up activity skyrocketing and new ventures thriving in the field. For example, according to this report, AI companies increased their activity by 72% in 2018 compared to 2017.
Deep learning has a big impact by using the paradigm of successful pattern matching in image identification. Since then, his success has expanded into other areas as well. Deep learning applications are widely used in business, commerce, and many other areas such as healthcare. As algorithms improve and hardware power increases, many new applications will emerge, and AI will become ubiquitous if it hasn't already. Many specific deep learning applications are outperforming humans today. For example, some time ago artificial intelligence surpassed humans. But for Go, a highly complex game that originated in China, it was considered unlikely that AI would beat the grandmasters for years to come. However, the artificial intelligence program AlphaGo, developed by Google DeepMind, defeated the reigning human champion in 2016. She achieved this by studying the actions of human experts and playing against herself many, many times. In essence, it was his teacher using a paradigm called reinforcement learning. This is a type of learning where he learns from his previous actions, playing against himself.
The Shortcomings of Deep Learning
Deep learning AI has a huge success, however, some experts are not sure if this pattern is enough for human-level intelligence. This technology has other problems as well. One of the disadvantages of ANNs is that they are extremely inadequate in explaining and transparency of their motives for making decisions. These are black-box architectures. This is especially problematic in applications such as health diagnostic systems, where practitioners need to understand their decision-making processes.
Understanding the arguments underlying the decisions can form the basis for explanations such as why the system is asking for input or "how the system arrived at its conclusions." Explanations are considered very important components of AI improvements. So much so that DARPA has for some time considered the current generation of AI technologies important for the future, but views their black nature as a major obstacle to the use of this technology. DARPA is a division of the US Department of Defense that researches new technologies. They say that the goal of these projects is to create tools that will allow the person deciding on the AI program to understand the reasons for the decision. I have already written about increasing the transparency of AI programs. Deep learning systems can sometimes make unpredictable decisions, so trusting these systems is critical to making them.
The AI Future
Participants in a recent survey were asked about the most disturbing concept about AI. The results were as expected: the participants were most concerned about the idea that the robot could harm them physically. Naturally, cars with close physical contact, such as self-driving cars and home managers, were seen as risky. However, when it comes to statistics, languages, personal assistants: people are more than willing to use AI in everyday tasks. According to the Royal Society, many of the potential social and economic benefits of technology depend on the environment in which they develop.
As data scientist Katie O'Neill wrote, algorithms are dangerous if they have scale, their work is secret, and their effects are devastating. Alison Powell, assistant professor at the London School of Economics, believes this mismatch between perceived and potential risk is common in new technologies. "This is part of the general problem of promising technology transfer: new technologies are so often positioned as 'personal' that it is difficult to perceive systematic risk."
AI is already being used to create devices that trick and trick human hackers. He quickly learns from our behavior, and humans create robots that are so human-like that they can be our lovers. AI also learns what is good and what is bad. Mark Ridl and Brent Harrison of Georgia Tech's School of Interactive Computing are leading a team that is trying to instill human ethics in AI through stories. Just like in real life we teach children about human values by reading stories to them, AI learns to distinguish bad from the good, bad from the good. Just as civilizations were built on a contract of expected behavior, we may need to develop an AI system that respects and fits into our social norms. Whatever robot or system we create, their decisions must be consistent with our ethical judgments.