What is Multimodal AI: Explanation and Examples
Jul 15, 2024 5660 seen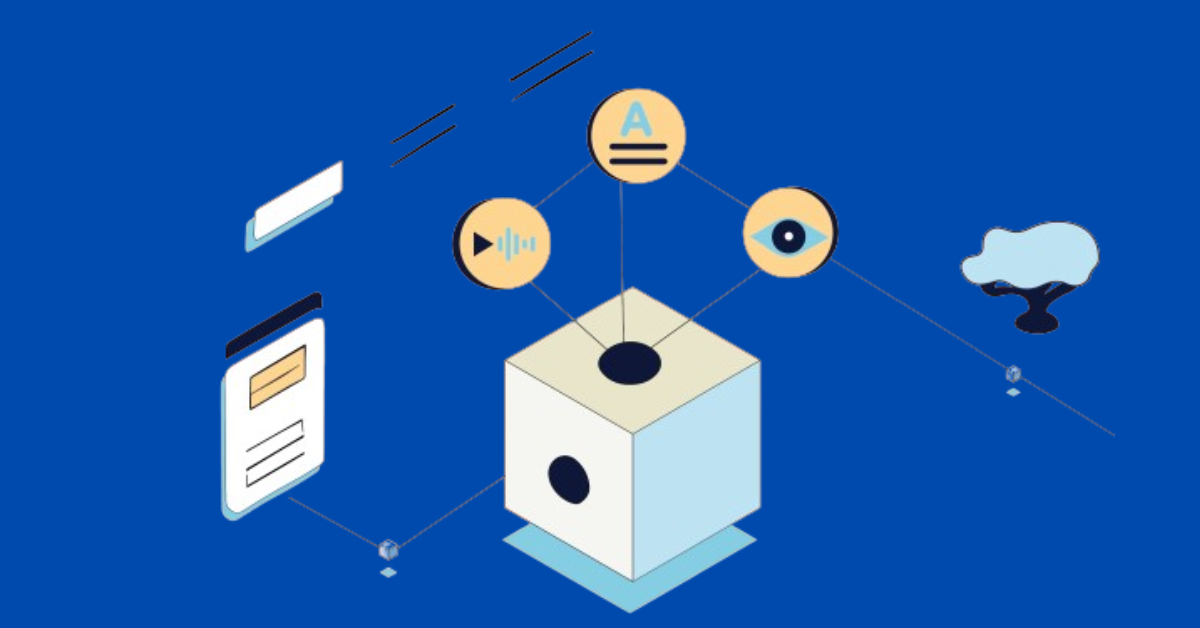
What is Multimodal AI Model
Are you familiar with multimodal AI models? If not, keep reading; we will review the topic and understand every detail.
The huge improvement in the AI industry is surely due to AI multimodal models, which provide unified processing and understanding of many kinds of data. The key feature is that many data formats, including text, photos, audio, and video, can be processed and understood by these models at the same time.
We will talk about the potential of multimodal AI models to revolutionize different industries by discussing their inner workings, applications, advantages, and disadvantages. Keep reading; I hope you'll enjoy it!
How Multimodal AI Models Work
Multimodal AI models use deep learning to connect characteristics from several modalities, integrating various data types into a coherent framework. To simplify it, let's have a look at particular examples:
- Data Collection and Preprocessing: The first step involves collecting various data types. For instance, a healthcare application might include medical images, patient records, and doctor's notes. Each data type is then preprocessed to make it suitable for the AI model.
- Feature Extraction: The model uses deep learning algorithms to extract relevant features from each data type. For example, in image processing, features might include shapes, colors, and textures, while in text processing, features could be keywords or sentiment.
- Data Integration: The extracted features are integrated into a unified representation. This step involves aligning data from different sources, ensuring that the temporal or contextual relationships between the data types are preserved.
- Training the Model: The integrated data is used to train the AI model. This involves feeding the data through neural networks, which learn to identify patterns and make predictions based on the combined information from multiple modalities.
- Inference and Prediction: Once trained, the model can make predictions or perform tasks by considering information from all available data types.
Examples of Where Can Be Used Multimodal AI Models
Ok, so now you know how multimodal AI works, it's time to understand where it can be used and how it can be beneficial for us:
- Healthcare: Multimodal AI can combine medical imaging data (like X-rays or MRIs) with patient records and genetic information to provide more accurate diagnoses and personalized treatment plans.
- Entertainment: In the entertainment industry, these models can enhance user experiences by integrating visual, auditory, and textual data to create more interactive and engaging content, such as virtual reality environments or personalized movie recommendations.
- Retail: Retailers can use multimodal AI to analyze customer behavior by integrating data from in-store video surveillance, online browsing patterns, and purchase history. This helps in creating personalized shopping experiences and targeted marketing campaigns.
- Autonomous Vehicles: In autonomous driving, multimodal AI models can process data from cameras, lidar, radar, and GPS to make real-time decisions. This comprehensive understanding of the environment enhances the safety and efficiency of self-driving cars.
- Virtual Assistants: Virtual assistants like Siri and Alexa can become more intuitive and responsive by integrating voice commands, contextual text data, and visual information from connected devices.
Challenges of Multimodal AI
As with every technology, multimodal AI faces significant challenges despite its benefits. Integrating different data types can be complex, requiring advanced techniques for alignment and synchronization. Besides, training these models demands substantial computational resources, including processing power and memory. Ensuring high-quality data from multiple sources is another hurdle, as inconsistent or incomplete data can hinder performance.
Lastly, interpreting how multimodal models make decisions presents challenges due to the complexity of integrating diverse data types. Addressing these challenges is crucial for fully realizing the potential of multimodal AI across various applications.